Interpreting model run results
In this document you will learn how to see the results of a Model Run using the Platform’s UI, interpret its KPIs, analyze the scores, and possible next steps on how to use and tweak the proposed planning solution.
Model run overview page
When a run has completed, the Model Run Overview page gives you a summary of the results from the run. Find this page by clicking the tile of a model, and then picking a run from the Run overview table.
The overview page has the following sections:
-
Sidebar on the right, with (in this order):
-
The run’s status.
-
The run’s KPIs.
-
The run’s properties.
-
-
Optionally, any error or warning messages.
-
The score graph and the hard, medium and soft scores.
-
The list of constraints with their score analysis.
Run status and errors/warnings
A run’s status is indicated at the top right in the sidebar.
Statuses include:
- Scheduled
-
The run is scheduled to start soon.
- Solving
-
The planning problem is currently being solved. During solving the UI will auto-update to reflect the current best solution.
- Completed
-
The run is finished, and completed successfully. The UI will reflect the best solution from the run.
- Failed
-
The run is finished, but there were problems.
If there were any errors or warnings related to the run (e.g. input validation) the overview page will show them.
Run KPIs
Each model defines their own KPIs. These are metrics that reflect the problem domain. KPIs give an indication of the quality of the provided solution.
Run properties
Below the run’s KPIs we show the other properties of the run:
-
The ID, the name, and tags that were provided at the start of the run.
-
The time the run was submitted, started, and completed.
-
The run’s score calculation speed. This is an indicator of how quickly Timefold is exploring different solutions and Timefold’s performance.
Score graph and scores
The score of a model run is an indication of its quality. The higher the score, the better the constraints are met, and the more optimal the provided solution is.
We distinguish between hard constraints, medium constraints, and soft constraints and compute scores for each.
- Hard constraints
-
Hard constraints are the basic rules of the domain and must never be broken. If they are broken, the solution isn’t even feasible.
- Medium constraints
-
Medium constraints usually incentivise Timefold to assign as many entities as possible. They are used by Timefold to allow for overconstrained planning.
- Soft constraints
-
The soft constraints of a model represent the optimization objectives. They can be broken, but the more they are satisfied, the more optimal a solution is.
Timefold optimizes for a higher hard constraint score first (to find a feasible solution), then a higher medium constraint score (to assign as much as possible), and then a higher soft constraint score (to optimize the solution). The scores are the sums of each of the constraint scores, grouped by type.
The graph below that shows the evolution of the scores for hard, medium, and soft constraints during the model run. You can click the expand button on the right of the chart to see each score on their own graph with Y-axis values.
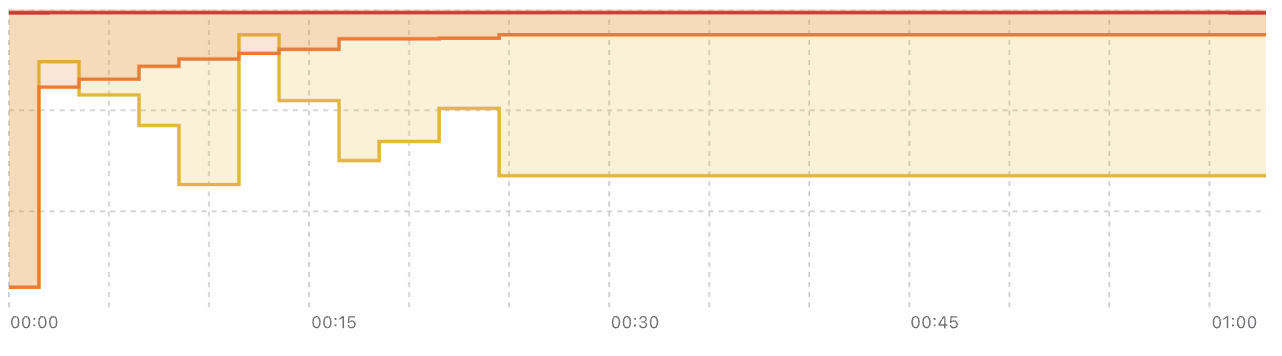
Score analysis and justification
Below the score graph is a list of all constraints defined by the model. The constraints that aren’t fully satisfied are presented first, ordered by type and then score.
By default, constraints that are fully met are hidden. Click Show satisfied constraints to reveal all constraints.
For each constraint we show:
-
Its name.
-
Its type: hard, medium or soft.
-
The impact: whether it’s a penalty or a reward.
-
The associated score.
For constraints that couldn’t be fully satisfied the constraint table indicates how often the constraint wasn’t met under Matches. By clicking on the arrow next to the constraint name, you can view the justifications that explain individual instances of the broken constraint.
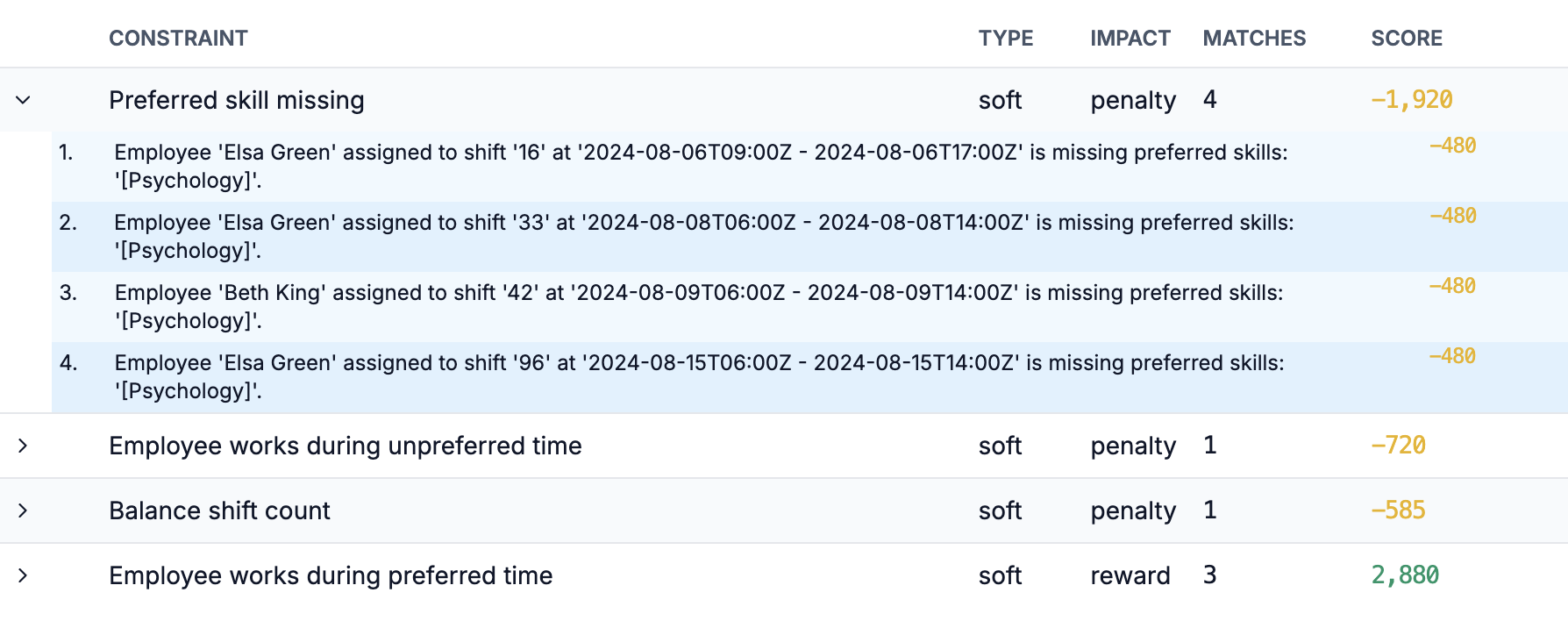
The image shows constraints that aren’t fully satisfied for a run of the employee scheduling model. Justifications are displayed for 4 instances. In each case, a preferred skill constraint wasn’t met, penalizing the soft constraint score by 480 points each. Additionally, the "Employee works during preferred time" constraint has three matches were the constrain was met and achieved a positive score (reward), rather than a penalty.
Planning solution output and visualization
A visual representation of this plan can be found on the Visualization page.
The full details of the solution can be found under Output. You can also download the output as a JSON file with the full details of the plan.
Using the API
The information from this overview page is also available by using the Model’s API.
-
The
/{id}
endpoint returns the best solution, including its KPIs. -
The
/{id}/run
endpoint returns the status of a run and any validation errors or warnings. -
The
/{id}/score-analysis
endpoint returns a list of the constraints, their scores, matches, and justifications.
For more information about the API endpoints, go to a model’s API Spec page.
What’s next? Tweaking the planning solution
Now that Timefold has provided you with an optimized plan for your planning problem, there are several ways you can further tailor the solution to your business needs.
Changing the optimization goal
When there is a feasible solution (meaning all hard constraints are met), Timefold further optimizes for soft constraints. By default each of these constraints are given the same importance, but you can give constraints different weights.
By giving a constraint a higher weight, you make it more important. For the field service routing model, you can use this to optimize more for travel time then for travel distance.
You can disable a constraint’s weight by setting it to 0.
Which combination of weights is right for your business depends on what you value most as a business. By using our platform, you can do multiple runs with different weights and then compare the KPIs to see or simulate the impact.
Look in the model’s API Spec for the config.model.overrides parameter for an overview
of which constraints can be adjusted this way.
|
Compare to other runs
The Model Runs Overview page, shows a table with the latest runs of a model. By default we show the scores of each of the runs, as well as the first KPIs. Use the search functionality to compare specific runs.
Click Manage columns to customize which columns are shown on the overview page. You can pick which of the model KPIs to compare. |
Plan around fixed segments
Timefold models allow you to pin certain segments, so you can fully customize a plan. Maybe there is an exception where you want to make sure a certain shift is done by a specific employee, or a certain visit is done by a specific vehicle. If you provide Timefold with pinned segments, it will honour those while planning around them.